Ai Curation
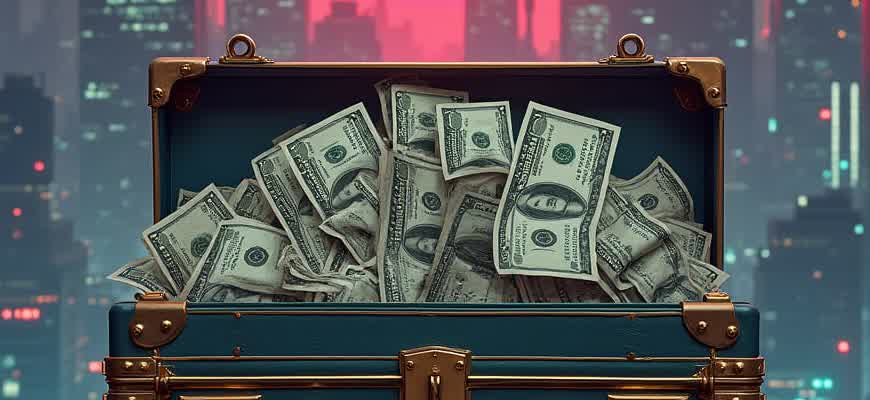
Artificial Intelligence is revolutionizing how content is curated, offering more personalized and efficient methods for selecting relevant material. By analyzing vast amounts of data, AI tools can identify patterns, preferences, and trends, which help to tailor content to individual users or specific audiences. This has applications across various industries, from marketing to education and entertainment.
One of the core methods for AI content curation involves machine learning algorithms that continuously learn from user interactions. These algorithms process data such as:
- Browsing behavior
- Search history
- Engagement metrics
By combining these inputs, AI models can predict what content a user is most likely to engage with, enhancing both user experience and content relevance.
Key Advantages of AI Curation:
Advantage | Explanation |
---|---|
Efficiency | AI quickly processes large amounts of data, reducing the need for manual curation. |
Personalization | AI delivers content that is specifically tailored to the preferences and behaviors of each user. |
"AI-powered curation systems are not just about automation; they enable a deeper connection between content and user interests, creating more impactful digital experiences."
AI-Driven Content Curation: Transforming the Way We Manage Digital Assets
The rapid evolution of artificial intelligence has paved the way for more efficient content management strategies. As organizations and individuals face an overwhelming influx of digital data, AI-powered curation tools have become essential in organizing, categorizing, and personalizing content. These tools not only streamline the management process but also help enhance user engagement by delivering more relevant and targeted material. By harnessing machine learning algorithms, AI is able to curate content at scale, offering insights that were previously time-consuming to uncover manually.
AI curation tools have the ability to analyze user behavior, understand preferences, and filter out irrelevant or redundant information. As a result, they contribute to building a more personalized content experience, which is crucial in today's fast-paced digital environment. This shift toward AI-driven content management has the potential to revolutionize industries such as marketing, media, and e-commerce, where the timely and relevant delivery of content plays a critical role in business success.
Key Benefits of AI Content Curation
- Efficiency and Time Savings: Automating the content curation process significantly reduces manual effort, allowing teams to focus on more strategic tasks.
- Personalization: AI can tailor content to individual users based on their preferences, behavior, and interactions, enhancing the overall user experience.
- Scalability: AI-driven systems can handle vast amounts of data, ensuring that content is curated and delivered across multiple platforms without loss of quality or relevance.
How AI Curation Works
- Data Collection: AI tools gather data from various sources, such as websites, social media platforms, and databases.
- Content Analysis: Machine learning algorithms analyze this data to identify patterns, trends, and key topics that resonate with the target audience.
- Content Delivery: Based on the analysis, AI curates and presents the most relevant content to users, adapting in real-time to their evolving preferences.
Applications of AI in Content Curation
Industry | Use Case |
---|---|
Marketing | Automated content suggestions for personalized campaigns |
Media | Recommendation systems for news articles and videos |
E-commerce | Product recommendations based on user browsing history |
AI-powered content curation is no longer a luxury but a necessity for businesses striving to remain competitive in the digital age. The future of content management is AI-driven, where relevance and personalization take center stage.
How AI Selects Content Based on Individual User Preferences
Artificial intelligence is increasingly used to tailor content to individual user tastes, improving engagement and user experience. This is achieved by analyzing a wide range of data points such as user behavior, interaction history, and demographic information. By processing vast amounts of data, AI systems can predict and deliver content that aligns with the specific interests of each user, making platforms more personalized and user-friendly.
The process of content curation involves multiple steps, from data collection to content recommendation. Machine learning algorithms are used to recognize patterns and preferences, allowing AI to make intelligent choices about what to present. Over time, the system learns and refines its recommendations, offering increasingly accurate and relevant content.
Key Factors Influencing AI Content Selection
- User Behavior: AI analyzes the user's clicks, likes, comments, and time spent on various pieces of content to identify preferences.
- Demographic Data: AI uses information such as age, location, and gender to deliver more targeted content.
- Content Interactions: The system evaluates past engagement with similar content to refine future recommendations.
How AI Learns and Improves Its Curation
- Data Collection: AI gathers data from user interactions, such as search queries and consumption patterns.
- Pattern Recognition: Algorithms identify trends and correlations within the collected data to predict user preferences.
- Continuous Feedback: AI adjusts its recommendations based on ongoing user interactions, ensuring that content remains relevant.
AI-based curation is a dynamic process that evolves with user behavior, ensuring that content recommendations stay fresh and relevant over time.
Example of AI Content Curation Process
Step | Description |
---|---|
Data Collection | AI gathers data on user behavior, preferences, and past interactions. |
Pattern Analysis | Machine learning algorithms analyze the data to uncover user trends. |
Content Recommendation | AI delivers personalized content based on identified preferences. |
Streamlining Workflow: Automating Content Selection with AI
In modern content curation, the task of filtering and selecting relevant material can be a time-consuming process, often requiring manual effort to sift through vast amounts of data. Leveraging AI technologies to automate this process allows businesses and content creators to optimize their workflow, making the selection process faster and more efficient. By utilizing advanced algorithms and machine learning models, AI can assess and prioritize content based on predefined criteria, such as user preferences, engagement metrics, or topical relevance.
Automation of content selection not only speeds up the workflow but also ensures consistency in the type and quality of content being curated. This becomes particularly useful in industries where content needs to be updated regularly and tailored to specific audiences. AI-powered systems can handle these tasks autonomously, freeing up time for creators to focus on higher-level strategic decisions.
Key Benefits of AI-Driven Content Curation
- Time Efficiency: Automated content selection significantly reduces the manual effort required for filtering and selecting relevant content.
- Consistency: AI ensures that content quality and thematic alignment remain consistent, avoiding human error or oversight.
- Personalization: Algorithms can customize content curation based on audience preferences and historical behavior, improving engagement.
How AI Enhances the Content Curation Process
- Data Collection: AI systems gather vast amounts of data from various sources such as social media, news websites, and industry blogs.
- Content Evaluation: The AI evaluates the data based on criteria like relevance, engagement, and freshness, prioritizing the most valuable content.
- Content Recommendation: AI suggests curated content for specific platforms or target audiences, improving the chances of content success.
"Automating the curation process not only saves time but also allows businesses to scale their content strategy without compromising on quality."
Example of AI-Driven Curation in Action
Step | AI Role | Outcome |
---|---|---|
Content Sourcing | AI crawls the web and aggregates data based on specific topics or keywords. | Quick identification of relevant content from a vast pool of information. |
Content Filtering | AI filters out irrelevant or low-quality content using predefined metrics. | Ensures only the most engaging and valuable content is curated. |
Content Delivery | AI automates the distribution of selected content to targeted channels. | Maximizes content reach and audience engagement. |
AI-Driven Personalization: Tailoring Content for Each Audience
AI technologies enable businesses to create deeply personalized user experiences by analyzing large sets of data and delivering targeted content that resonates with each individual. By employing machine learning algorithms, companies can understand user preferences, behaviors, and needs, allowing for a more customized interaction. This level of personalization leads to higher engagement rates and greater user satisfaction, as the content becomes relevant to each person.
With AI, content curation is no longer a one-size-fits-all approach. Instead, AI systems dynamically adjust content based on various factors such as user location, browsing history, and interaction patterns. This ensures that content is tailored to provide the most value, creating a more engaging and meaningful experience for each audience segment.
Key Components of AI-Powered Content Personalization
- Behavioral Data Analysis: AI systems track user actions to understand preferences and adjust content accordingly.
- Real-Time Adaptation: Content is continuously updated to reflect the most current user needs and interests.
- Predictive Analytics: AI predicts what content the user may be interested in based on past behavior and trends.
Methods for Effective Personalization
- Segmentation: Grouping users by demographics, behavior, or interests to deliver content that is specific to each group.
- Dynamic Content Delivery: Content is modified in real-time based on user inputs and interaction history.
- Recommendation Engines: AI suggests new content based on previous choices and preferences.
"Personalization is not about delivering the same content to everyone, but about delivering the right content to the right person at the right time."
Content Personalization Metrics
Metric | Description | Impact |
---|---|---|
Click-Through Rate (CTR) | Measures the effectiveness of personalized content in prompting user actions. | Higher CTR indicates more relevant content and higher user engagement. |
Conversion Rate | Tracks the percentage of users taking the desired action, such as making a purchase. | Improved conversion rates reflect stronger alignment between content and user needs. |
Time on Page | Measures the amount of time users spend interacting with personalized content. | Longer time on page suggests higher content relevance and engagement. |
Reducing Information Overload: How AI Filters Out Irrelevant Content
In the era of constant digital interaction, the volume of information available to us has grown exponentially. This has led to a situation where individuals are bombarded with more data than they can process. As a result, effectively managing and sorting relevant from irrelevant information has become a crucial challenge. AI plays a key role in mitigating this overload by automating the process of filtering and organizing vast amounts of content based on specific relevance criteria.
By utilizing machine learning and natural language processing, AI algorithms can discern patterns in user behavior, preferences, and context to prioritize the most pertinent data. This dynamic filtering allows AI systems to not only sort content but also to predict the most useful information before it reaches the user. Below are some of the key mechanisms AI uses to filter out unnecessary content:
AI Methods for Filtering Irrelevant Information
- Personalization: AI tailors content by analyzing past behavior and preferences.
- Contextual Understanding: AI evaluates content in relation to the user's immediate needs and context.
- Data Clustering: AI groups related content, reducing the exposure to unrelated information.
- Sentiment Analysis: AI filters out content that may be irrelevant due to negative sentiment or poor quality.
These methods help reduce cognitive load and increase content relevance for the user. AI’s ability to continually refine its understanding of what matters most ensures that irrelevant data is filtered with greater accuracy over time.
"AI’s filtering process evolves through continuous learning, allowing it to anticipate user needs and tailor information delivery more precisely."
AI in Practice: Examples and Benefits
Here are some practical examples where AI’s filtering capabilities significantly reduce information overload:
- News Aggregation: AI-powered apps sort news articles based on user interests and the credibility of sources.
- Social Media Feeds: Algorithms curate content to show only what is most relevant to the user’s preferences and interactions.
- Email Management: AI systems help sort emails by importance, reducing distractions and focusing attention on key communications.
These applications highlight how AI’s filtering abilities streamline information consumption, improving efficiency and user experience.
AI Filtering Method | Application | Benefit |
---|---|---|
Personalization | News Aggregators | Shows only relevant articles, reducing irrelevant news clutter. |
Data Clustering | Social Media Feeds | Groups similar posts, making content easier to process. |
Sentiment Analysis | Email Management | Prioritizes important emails by assessing tone and urgency. |
Improving Engagement Through Smart Content Recommendations
Effective content curation plays a crucial role in maintaining audience interest and driving engagement across digital platforms. By leveraging AI-powered systems, brands can enhance user experiences by delivering personalized and relevant content that aligns with individual preferences and behaviors. This dynamic approach not only improves engagement but also boosts user retention and satisfaction.
AI-driven content recommendation systems utilize complex algorithms to analyze user activity, preferences, and historical data, suggesting content that is most likely to resonate with the user. These systems continuously learn from user interactions, making them increasingly effective over time.
Key Benefits of Smart Content Curation
- Personalized Experience: AI tools tailor content recommendations to individual users, ensuring that each piece of content feels relevant.
- Increased Engagement: By offering content that directly matches user interests, engagement rates such as click-through and conversion rates are significantly improved.
- Optimized Discovery: Users are more likely to discover new content they find valuable, keeping them engaged for longer periods.
How AI Algorithms Improve Content Recommendations
- Data Collection: The system tracks and analyzes user behaviors such as clicks, time spent, and interactions with content.
- Pattern Recognition: AI identifies patterns in user activity, allowing it to predict what type of content a user is most likely to engage with.
- Continuous Learning: The AI continually updates its models based on new data, improving accuracy and content relevance.
Impact on User Retention
"AI-driven content recommendations provide users with a seamless and engaging experience, increasing the likelihood of their return to the platform."
Example of AI-Driven Content in Action
Platform | AI Feature | Impact |
---|---|---|
Netflix | Personalized movie suggestions | Increased viewing time and user retention |
YouTube | Recommended videos based on watch history | Improved viewer engagement and content discovery |
Assessing the Effect of AI-Driven Content Curation on User Engagement
Content curation through AI has transformed how platforms retain users by delivering highly personalized and relevant material. By utilizing machine learning algorithms, AI can analyze vast amounts of data, identifying trends and preferences specific to each user. This level of personalization can result in improved user satisfaction and longer interaction times, which are critical factors for retention.
To effectively measure the impact of AI content curation on user retention, various metrics should be considered. These include user session length, return frequency, and overall engagement with curated content. Additionally, monitoring how well AI-generated recommendations align with user preferences over time is essential to evaluate its effectiveness in retaining a dedicated user base.
Key Metrics for Evaluation
- Session Duration: Measures how long users stay on the platform after engaging with AI-curated content.
- Return Rate: Tracks how often users return to the platform after initial interactions with personalized content.
- Engagement Levels: Assesses how users interact with AI-generated recommendations, including likes, shares, and comments.
Impact on User Retention
Studies show that when users feel content is tailored to their interests, their likelihood of returning to the platform increases by 30%.
The key to improving retention lies in consistently refining the curation algorithms based on user feedback and behavioral data. AI systems must adapt to evolving preferences, ensuring that the content remains relevant and engaging. Regular analysis of user interactions provides valuable insights for optimizing AI models to keep content fresh and aligned with user expectations.
Data-driven Insights
Metric | Before AI Curation | After AI Curation |
---|---|---|
Average Session Duration | 5 minutes | 12 minutes |
Return Frequency | 2 times per week | 5 times per week |
User Engagement | Low | High |
Integrating AI Curation Tools with Your Existing Platforms
AI curation tools offer significant advantages in organizing and personalizing content, but integrating them into your current systems requires thoughtful planning and technical alignment. Proper integration not only enhances the user experience but also boosts efficiency and relevance by leveraging AI's ability to analyze and adapt to user preferences. However, the process of seamless integration with existing platforms, whether it's a content management system (CMS) or an e-commerce website, involves addressing several technical and operational challenges.
Successful integration involves aligning the AI curation capabilities with the specific needs of your platform. This requires a clear understanding of the platform’s architecture and the intended outcomes, whether it's improving user engagement, content discovery, or optimizing recommendations. Below are key considerations when integrating AI curation tools.
Key Steps for Integration
- Assess the Compatibility: Before implementation, ensure the AI curation tool is compatible with your platform's technology stack, APIs, and data protocols.
- Data Synchronization: Efficient data flow is crucial for accurate recommendations. Make sure the tool has access to the necessary data sources like user behavior, preferences, or previous interactions.
- Customization of Algorithms: AI models should be tailored to the specific needs of your platform to ensure that recommendations align with your goals and user expectations.
Implementation Challenges
Integration of AI tools can be challenging due to the complexity of aligning AI algorithms with pre-existing systems, data consistency, and ensuring that user privacy is respected.
Common implementation hurdles include data quality issues, system architecture differences, and the need for continuous monitoring and fine-tuning. Additionally, ensuring that AI systems are transparent and comply with privacy regulations is vital for maintaining user trust and adhering to legal requirements.
Benefits of AI Integration
- Personalized Content Delivery: AI-driven recommendations improve user engagement by offering more relevant and customized content.
- Increased Efficiency: AI tools automate curation processes, reducing the manual effort required and speeding up decision-making.
- Scalable Solutions: As your platform grows, AI curation tools can scale effortlessly to handle larger volumes of data and users.
Example Integration: E-commerce and AI Curation
Platform | AI Tool | Integration Goal |
---|---|---|
E-commerce Website | Personalized Product Recommendations | Increase conversion rate by displaying products based on customer behavior and preferences |
Content Management System | Content Personalization | Deliver articles, blogs, and media that match user interests |
Overcoming Common Challenges in AI Content Curation Implementation
Implementing AI-driven content curation can bring significant efficiency and relevance to digital content management. However, several obstacles often arise during deployment, which can hinder its success. These challenges range from data quality issues to algorithmic biases, all of which need to be addressed for AI curation to be truly effective. A deep understanding of these hurdles and practical strategies for overcoming them can help organizations better integrate AI tools into their content processes.
One of the major difficulties is ensuring the AI system is trained on high-quality, diverse data. Insufficient or biased data can lead to poor content recommendations or even reinforce stereotypes. Another challenge lies in aligning AI-driven curation with human editorial standards. Maintaining a balance between automation and human oversight is crucial to ensure that AI recommendations resonate with target audiences while preserving content authenticity.
Key Challenges and Solutions
- Data Quality and Bias
- AI models heavily rely on data, and if this data is unrepresentative or biased, the output will be flawed. This can lead to content recommendations that may alienate certain user groups.
- To overcome this, companies must invest in gathering diverse, high-quality datasets and continually refine their models based on feedback.
- Integration with Existing Systems
- Incorporating AI content curation into existing content management systems can be complex and costly, often requiring significant infrastructure changes.
- A practical solution is to use flexible, modular AI tools that can be easily integrated into current workflows, minimizing disruption and ensuring smooth transitions.
- Maintaining Editorial Control
- AI-powered systems may suggest content that lacks human intuition, cultural relevance, or emotional resonance with the audience.
- Establishing oversight processes where content curation algorithms are reviewed periodically by human editors is an essential step in ensuring quality and relevance.
"To ensure AI content curation is successful, it is important to continually monitor its performance and adapt to evolving trends and audience preferences."
Practical Steps for Overcoming Challenges
- Invest in Data Diversity – Ensure the AI model is exposed to a wide range of data sources to reduce the risk of bias.
- Implement Hybrid Models – Combine AI-generated recommendations with human editorial input to maintain relevance and accuracy.
- Monitor and Refine Algorithms – Regularly update and test algorithms to keep them aligned with current trends and user needs.
Challenge | Solution |
---|---|
Data Quality | Gather diverse and unbiased datasets; continuously refine the models based on real-time feedback. |
Integration with Existing Systems | Choose modular AI tools that seamlessly integrate with current platforms to reduce friction. |
Editorial Control | Implement hybrid content curation models and periodic human review processes. |