Ai Traffic Controller
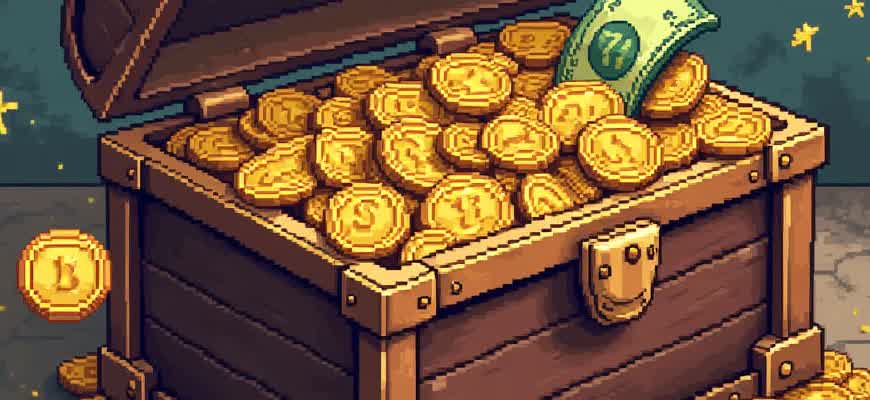
The integration of artificial intelligence into urban traffic control systems represents a significant leap forward in transportation technology. AI traffic controllers are designed to optimize the flow of vehicles, reduce congestion, and improve safety by using real-time data and advanced algorithms. These systems rely on a combination of machine learning, sensor networks, and predictive analytics to make autonomous decisions on traffic light patterns, lane usage, and incident management.
Key Benefits:
- Increased Traffic Flow Efficiency
- Reduction in Traffic Accidents
- Real-time Incident Detection
- Lower Environmental Impact due to Optimized Traffic
How It Works:
- Data is collected from sensors embedded in the road infrastructure.
- AI algorithms analyze the traffic patterns and adjust signal timings accordingly.
- Incidents or unusual traffic behaviors are detected, triggering real-time adjustments to routes and signals.
- The system continuously learns and improves from past traffic data to enhance future predictions.
"AI traffic controllers are not just about automating signal changes; they represent a shift towards a dynamic, adaptive traffic management system that can respond to real-time conditions."
Comparison of Traditional vs. AI Traffic Management:
Aspect | Traditional Systems | AI-Driven Systems |
---|---|---|
Traffic Flow Adjustment | Fixed signal timings | Dynamic, based on real-time data |
Incident Management | Manual intervention | Automatic detection and response |
Environmental Impact | High emissions due to inefficient traffic flow | Lower emissions from optimized traffic management |
AI Traffic Controller: Revolutionizing Traffic Flow Management
The integration of Artificial Intelligence (AI) into traffic management systems is poised to transform the way urban areas handle vehicle flow. Traditional traffic control methods, primarily based on static traffic light schedules and human intervention, often lead to congestion, delays, and inefficiencies. AI-powered systems are designed to adapt to real-time traffic conditions, making dynamic adjustments that optimize the movement of vehicles across cities.
AI traffic controllers use complex algorithms that process data from a variety of sensors, cameras, and IoT devices, allowing them to make decisions based on live traffic patterns. These systems not only improve the speed of traffic but also reduce accidents and environmental impacts by minimizing idle times at intersections.
Key Features of AI-Driven Traffic Management
- Real-time Data Processing: AI systems continuously monitor traffic through sensors, cameras, and GPS data from vehicles.
- Adaptive Signal Timing: Traffic lights are adjusted dynamically based on traffic flow, reducing unnecessary delays.
- Accident Prevention: AI can predict potential accidents and alert drivers or adjust signals to prevent collisions.
- Environmental Impact: By reducing idling time, AI helps to cut down on fuel consumption and emissions.
Advantages of Implementing AI in Traffic Control
- Improved Traffic Flow: AI systems can optimize green light times and reduce bottlenecks, improving overall traffic efficiency.
- Cost Savings: AI reduces the need for manual traffic control and lowers operational costs in the long term.
- Increased Safety: AI can detect hazardous conditions or accidents and make rapid adjustments to prevent further incidents.
Impact on Future Urban Mobility
Metric | Before AI Integration | After AI Integration |
---|---|---|
Traffic Congestion | High | Low |
Average Commute Time | Long | Short |
Carbon Emissions | High | Reduced |
"AI is not just a tool for the future, but a game-changer in modernizing the way we approach traffic control today."
How AI-Driven Traffic Controllers Enhance Real-Time Traffic Management
Artificial Intelligence (AI) plays a pivotal role in modern traffic systems, revolutionizing how traffic flows in real-time. Unlike traditional methods, AI traffic controllers use machine learning algorithms to predict traffic patterns and optimize the flow of vehicles through intersections. This reduces congestion and improves overall driving efficiency, minimizing wait times at signals and enhancing safety for all road users.
AI systems continuously process data from cameras, sensors, and traffic signals to adapt to changing conditions. This dynamic approach ensures that traffic light timing is adjusted in real-time based on the current traffic load, weather conditions, and even special events. The ability to make these adjustments without human intervention significantly increases the system's responsiveness and adaptability.
Key Benefits of AI Traffic Controllers
- Real-Time Traffic Flow Optimization: AI controllers adjust signal timings to ensure smoother traffic transitions, reducing idle times and enhancing vehicle throughput.
- Dynamic Adaptation: The system responds instantly to changing traffic volumes, accidents, or roadwork, minimizing delays and rerouting traffic as needed.
- Reduced Environmental Impact: By reducing stop-and-go driving, AI helps decrease fuel consumption and emissions.
How AI Systems Manage Traffic in Real-Time
- Data Collection: Sensors and cameras collect data about traffic flow, vehicle speed, and congestion.
- Data Processing: AI algorithms analyze the data to detect patterns, predict traffic spikes, and assess optimal signal timings.
- Decision Making: AI adjusts traffic signals based on real-time data, ensuring efficient flow without manual intervention.
- Feedback Loop: AI continuously learns from outcomes, refining its algorithms to improve future decisions.
“AI traffic controllers do not only optimize existing traffic flow but are capable of predicting and managing unexpected traffic scenarios, which greatly enhances urban mobility.”
Comparison of Traditional vs AI-Based Traffic Control
Feature | Traditional Traffic Control | AI-Based Traffic Control |
---|---|---|
Adaptability | Fixed timing schedules | Dynamic, real-time adjustments |
Data Sources | Manual observations, basic sensors | Advanced sensors, cameras, and machine learning models |
Efficiency | Limited by pre-set schedules | Highly efficient, reduces delays and congestion |
Optimizing Traffic Signals with AI: A Step-by-Step Approach
Modern urban areas face increasing challenges in managing traffic efficiently. As traffic volume grows, traditional traffic signal systems become less effective in adapting to real-time conditions. Artificial intelligence offers a promising solution to optimize these signals, improving traffic flow and reducing congestion. By leveraging AI, cities can implement dynamic traffic control that adjusts to varying traffic conditions, weather, and accidents.
The key to AI-driven traffic signal optimization lies in continuous data collection and real-time decision-making. Through the use of sensors, cameras, and traffic data, AI systems can predict traffic patterns, identify bottlenecks, and adjust light timings accordingly. This process ensures smoother traffic movement, decreases wait times, and reduces fuel consumption, benefiting both drivers and the environment.
Step-by-Step Process for Optimizing Traffic Signals
- Data Collection: Traffic data is collected using sensors, cameras, and IoT devices placed on roads, at intersections, and along highways.
- Data Analysis: AI algorithms analyze the gathered data to identify trends, congestion points, and patterns in real-time traffic flow.
- Signal Adjustment: Based on the analysis, AI adjusts signal timings dynamically to alleviate traffic jams, ensure smooth vehicle flow, and reduce waiting times.
- Continuous Monitoring and Learning: The system continuously monitors traffic conditions and improves its decision-making by learning from past traffic patterns and adjusting its models accordingly.
"AI-enhanced traffic systems can reduce waiting times by up to 25% and cut down on overall traffic congestion, leading to faster commutes and improved urban mobility."
Key Benefits of AI-Driven Traffic Management
Benefit | Description |
---|---|
Reduced Traffic Congestion | AI adjusts signals in real-time, reducing traffic jams and improving overall flow. |
Improved Safety | By reducing traffic gridlock, AI reduces the likelihood of accidents caused by abrupt stops and starts. |
Environmental Impact | With optimized traffic flow, fuel consumption is reduced, lowering emissions and enhancing sustainability. |
By integrating AI into traffic signal systems, cities can create smarter, more efficient urban environments. This technology not only improves daily commutes but also contributes to long-term sustainability goals by reducing emissions and optimizing traffic infrastructure.
Reducing Congestion: AI's Role in Dynamic Route Adjustments
Traffic congestion has become a persistent issue in urban environments, negatively impacting commuting times and fuel consumption. As cities grow and the number of vehicles increases, the static nature of traditional traffic management systems proves inefficient in handling the dynamic flow of traffic. AI-powered systems are emerging as a solution to optimize road usage and enhance traffic flow, offering a more adaptive approach to managing congestion. By leveraging real-time data, AI can analyze traffic patterns and adjust routes dynamically, ensuring smoother journeys for drivers and minimizing delays.
One of the key advantages of AI in traffic management is its ability to process vast amounts of data from various sources, such as sensors, cameras, and GPS systems, to make informed decisions. Through machine learning algorithms, AI can predict traffic conditions, identify bottlenecks, and suggest alternative routes in real-time. This approach goes beyond traditional traffic lights and fixed-route systems, providing a flexible and intelligent solution to urban transportation challenges.
Dynamic Route Adjustments through AI
- Real-time Traffic Analysis: AI continuously processes data from traffic sensors, GPS, and social media to monitor traffic flow and detect anomalies like accidents or road closures.
- Predictive Traffic Modeling: AI uses historical data and real-time inputs to forecast future traffic patterns, allowing for the proactive adjustment of routes before congestion occurs.
- Adaptive Traffic Signals: AI-based systems can control traffic light timings based on current traffic volume, optimizing the flow of vehicles across multiple intersections.
"AI’s ability to adapt to traffic conditions in real-time ensures that routes can be optimized for every driver, reducing unnecessary delays and improving overall traffic management."
- Improved Route Planning: AI systems suggest alternate paths to avoid congestion, helping drivers save time and fuel.
- Reduced Congestion Zones: By distributing traffic evenly across available routes, AI can alleviate overcrowded roads and decrease bottlenecks.
- Enhanced Public Transit Coordination: AI helps optimize routes not only for private vehicles but also for buses and other forms of public transport, ensuring efficient citywide mobility.
AI Traffic Solution | Impact on Congestion |
---|---|
Predictive Traffic Modeling | Reduces wait times by forecasting and managing traffic flow before congestion peaks. |
Dynamic Route Suggestions | Distributes traffic more evenly, preventing overloading of specific routes. |
Adaptive Traffic Signals | Optimizes signal timings in real-time to maintain consistent traffic flow. |
Integrating AI-Based Traffic Management Systems with Current Infrastructure
Integrating AI-driven traffic controllers into existing road management systems presents both challenges and opportunities. The primary goal of this integration is to improve the efficiency of traffic flow while minimizing disruptions to the current infrastructure. This can be achieved through strategic adaptations to sensors, communication networks, and control algorithms. While the potential benefits of AI are vast, careful planning is needed to ensure compatibility with the current traffic environment, which may include outdated or incompatible technologies.
To ensure a smooth transition from traditional to AI-based systems, collaboration between AI developers, traffic authorities, and infrastructure engineers is essential. AI controllers rely heavily on real-time data, so the infrastructure must be equipped to handle and transmit this information efficiently. The existing infrastructure may require upgrades in terms of sensor coverage, communication bandwidth, and data processing capabilities to fully leverage the capabilities of AI-driven systems.
Key Considerations for Integration
- Compatibility with Legacy Systems: AI traffic controllers must be designed to work with existing traffic light systems, sensors, and cameras, which may not support the latest technologies.
- Real-Time Data Processing: The integration should prioritize fast data collection and processing. AI systems rely on vast amounts of data to make timely decisions, which may require upgrading communication networks.
- Scalability: Solutions should be scalable, allowing for easy expansion or adjustment as traffic demands change or as new technologies are introduced.
"Successful integration requires continuous monitoring and refinement of AI algorithms, as the system will need to adapt to varying traffic conditions and user behaviors over time."
Steps for Smooth Integration
- Conduct a thorough audit of the existing infrastructure to identify gaps in sensors, communication networks, and control systems.
- Install or upgrade necessary sensors and cameras to ensure real-time data availability and accurate decision-making by the AI system.
- Develop custom algorithms that can be retrofitted to the current traffic management platform while ensuring minimal disruption to existing operations.
- Implement a phased rollout plan to gradually introduce AI controllers into the network, allowing for testing and adjustments along the way.
Infrastructure Requirements
Infrastructure Component | AI Integration Requirement |
---|---|
Sensors and Cameras | Upgrade to support high-definition, real-time data collection for AI algorithms |
Communication Networks | Increase bandwidth and ensure low-latency connections for data transmission |
Traffic Control Systems | Ensure compatibility or upgrade to handle AI-driven decision-making |
Cost Savings for Municipalities Through AI-Driven Traffic Systems
AI-driven traffic management systems can significantly reduce operational costs for municipalities by optimizing traffic flow and resource allocation. By leveraging machine learning and real-time data analysis, these systems are able to minimize traffic congestion, leading to lower fuel consumption and reduced wear and tear on infrastructure. In addition, automation allows for more efficient use of manpower, reducing the need for constant human oversight and intervention. These savings can be reinvested into other municipal services, enhancing the overall efficiency of the local government.
Furthermore, AI systems contribute to long-term cost reduction by predicting traffic patterns and planning for future infrastructure needs. This predictive capability helps municipalities avoid costly overbuilds or unnecessary expansions, allowing for more targeted investment in road maintenance and public transportation improvements. With these advancements, cities can operate at lower costs while providing better services to residents.
Key Areas of Cost Savings
- Reduced Fuel Consumption: Traffic optimization leads to fewer traffic jams, saving fuel for both public and private vehicles.
- Lower Maintenance Costs: Predictive maintenance of infrastructure based on AI analysis extends the lifespan of roads and bridges.
- Improved Resource Allocation: AI can allocate traffic management resources more effectively, reducing the need for manual intervention.
AI Impact on Traffic Management Costs
Cost Area | Before AI | After AI | Annual Savings |
---|---|---|---|
Fuel Costs | High due to traffic congestion | Reduced due to optimized traffic flow | Up to 20% |
Maintenance Costs | Frequent repairs and updates | Longer lifespan due to predictive analysis | Up to 15% |
Personnel Costs | High due to manual monitoring | Reduced with automated systems | Up to 30% |
Important: AI traffic management systems not only save costs but also improve the quality of life for residents by reducing travel times and lowering stress levels on the road.
How AI Traffic Controllers Adapt to Peak Traffic Times
AI-based traffic management systems are designed to handle high traffic loads by utilizing data analytics, machine learning, and real-time monitoring to optimize traffic flow. During peak hours, these systems become even more essential as they need to adjust dynamically to the changing conditions on the roads. By continuously analyzing traffic patterns and vehicle density, AI controllers can predict and respond to congestion efficiently, minimizing delays and improving overall traffic management.
The ability of AI traffic controllers to adapt to peak times is largely driven by their capacity to process large amounts of data in real-time. These systems use sensors, cameras, and traffic signals to collect data, which is then processed to adjust traffic lights, speed limits, and other traffic control measures. The goal is to reduce traffic jams, improve safety, and optimize fuel efficiency for all road users.
Key Strategies Employed by AI Traffic Controllers
- Real-time Traffic Analysis: AI systems constantly monitor traffic flow and detect anomalies in real-time. When traffic congestion is detected, the system adjusts traffic signals to prioritize heavy flows of vehicles.
- Predictive Adjustments: Using historical data and machine learning algorithms, AI controllers can predict peak times and adjust the traffic flow accordingly before congestion occurs.
- Dynamic Signal Adjustments: Traffic lights are not fixed but change based on real-time data, adjusting to traffic density and speed to avoid bottlenecks.
Adaptation Process at Peak Hours
- Data Collection: AI systems collect data from a variety of sensors and cameras installed throughout the city.
- Real-Time Analysis: The collected data is immediately analyzed to detect traffic patterns, identify congested areas, and determine the necessary adjustments.
- Traffic Light Coordination: Signals are adjusted to allow smooth flow through intersections, prioritizing high-volume routes.
- Feedback Loop: The system continuously monitors the effectiveness of adjustments and recalibrates in response to changing traffic conditions.
Example of AI Traffic Control Performance
Time Period | Average Traffic Flow | Average Wait Time | System Adjustment |
---|---|---|---|
Morning Rush | 80% capacity | 5-7 minutes | Increased green light duration |
Midday | 60% capacity | 2-3 minutes | Standard timings maintained |
Evening Rush | 95% capacity | 8-10 minutes | Real-time rerouting suggestions |
AI traffic controllers use predictive algorithms to anticipate peak times and proactively adjust traffic signals, ensuring minimal disruption during high-traffic periods.
The Influence of AI-Based Traffic Systems on Reducing Accidents
Artificial Intelligence (AI) is reshaping the way cities manage traffic, bringing about significant improvements in safety. By using advanced algorithms to monitor and control traffic flow, AI systems are able to predict potential hazards and respond in real-time to reduce the risk of accidents. These systems analyze data from various sources such as cameras, sensors, and GPS to optimize traffic light timings, manage congestion, and even detect unsafe driving behaviors.
AI-powered traffic management offers a proactive approach to accident prevention. By adjusting traffic flow dynamically and providing real-time information to drivers, AI minimizes the chances of accidents caused by human error, such as speeding, running red lights, or failing to yield. These technologies also facilitate smoother transitions between intersections and reduce the likelihood of collisions, particularly in areas with high traffic volumes.
How AI Reduces Traffic Accidents
- Real-time hazard detection: AI systems continuously monitor the road environment, identifying sudden changes like stopped vehicles or erratic driver behavior.
- Optimized signal control: AI adjusts traffic light sequences based on traffic conditions, ensuring vehicles move efficiently and safely.
- Driver alerts: AI-based systems can send warnings to drivers about upcoming hazards or unsafe driving conditions, promoting safer driving behavior.
- Intelligent route planning: By analyzing traffic patterns, AI can suggest alternative routes, reducing congestion and the potential for accidents.
Effectiveness in Accident Reduction
"Studies have shown that cities implementing AI traffic management systems experience a significant decrease in accidents, with some areas reporting up to a 30% reduction in collisions."
One of the major advantages of AI in traffic management is its ability to analyze vast amounts of data in real time and respond instantaneously. Traditional traffic management systems often rely on fixed schedules, which can fail to address rapidly changing traffic conditions. In contrast, AI can adapt to fluctuations in traffic volume, weather conditions, and accidents, ensuring a safer driving environment.
Feature | Impact on Safety |
---|---|
Predictive Traffic Modeling | Prevents accidents by anticipating traffic congestion and rerouting vehicles. |
Real-Time Incident Detection | Reduces response times to accidents, lowering the chances of secondary collisions. |
Driver Behavior Monitoring | Alerts drivers to dangerous actions like speeding, reducing the risk of accidents. |