Ai Driven Personalization
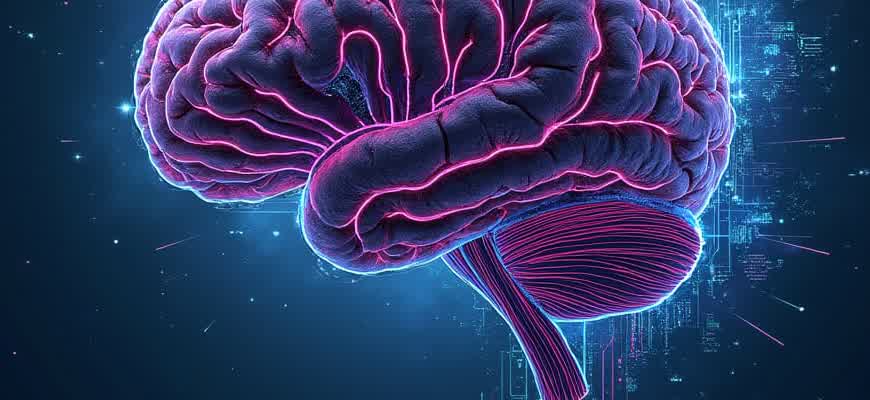
Artificial intelligence (AI) is revolutionizing the way businesses interact with their customers by providing highly customized experiences. AI algorithms analyze user behavior, preferences, and interactions to deliver personalized content that enhances engagement. This approach enables brands to tailor their services in real-time, making the customer journey smoother and more relevant.
Key aspects of AI-driven personalization:
- Data-Driven Insights: AI gathers and processes vast amounts of data, identifying patterns in user behavior.
- Real-Time Customization: AI systems adjust content dynamically, based on the user’s actions and preferences.
- Improved Engagement: Personalized recommendations and tailored experiences increase user satisfaction and retention.
"AI allows businesses to anticipate user needs and deliver content that feels uniquely suited to each individual, creating deeper connections."
AI-Powered Personalization Strategies:
- Recommendation Systems: AI models predict what a user might be interested in based on past interactions.
- Targeted Advertising: Ads are personalized to match a user's interests, improving conversion rates.
- Personalized User Interfaces: Interfaces are dynamically adjusted to meet the user’s needs and preferences.
AI Personalization Metrics:
Metric | Impact |
---|---|
User Retention | Increased due to relevant content and engagement strategies. |
Conversion Rate | Higher with personalized recommendations and targeted ads. |
Customer Satisfaction | Improved through individualized experiences. |
AI-Driven Personalization: A Practical Guide
Artificial Intelligence has revolutionized the way businesses tailor their services and products to individual customers. By analyzing large datasets and learning from user interactions, AI can deliver a highly personalized experience, from content recommendations to dynamic pricing strategies. However, implementing AI-driven personalization requires careful planning and the right set of tools to ensure it aligns with both business goals and customer needs.
This guide will walk you through the essential components of AI-driven personalization and the steps needed to implement it effectively. From understanding user behavior to choosing the right machine learning models, this approach enables businesses to foster stronger customer relationships and improve overall engagement.
Key Steps in Implementing AI Personalization
- Data Collection: Gather relevant customer data across various touchpoints. This includes behavioral data, purchase history, demographic information, and engagement metrics.
- Data Processing: Clean and process the data to ensure it is structured and ready for analysis. This step often involves data normalization and handling missing values.
- Model Selection: Choose the appropriate machine learning model based on your business requirements. Popular choices include collaborative filtering, decision trees, and neural networks.
- Personalized Content Delivery: Use the trained models to create dynamic, individualized content, product recommendations, or promotional offers that cater to each user’s preferences.
- Continuous Monitoring: Regularly evaluate the effectiveness of the AI-driven system and adjust the models to improve performance based on new data.
“AI-driven personalization is not a one-time implementation, but an ongoing process that evolves with customer behavior and business objectives.”
Best Practices for Effective AI Personalization
- Be Transparent: Let customers know how their data is being used. This builds trust and helps with compliance, especially in regions with strict data privacy regulations.
- Use Segmentation Wisely: While personalization can be highly effective, over-segmentation can lead to missing out on opportunities. Find the right balance between generalization and hyper-personalization.
- Test and Iterate: Personalization strategies should be constantly tested and refined based on real-time feedback and analytics.
Metrics to Track AI Personalization Success
Metric | Description | Why It Matters |
---|---|---|
Customer Retention Rate | The percentage of customers who return to your platform. | Indicates if personalized experiences are driving customer loyalty. |
Conversion Rate | The percentage of visitors who make a purchase or complete a desired action. | Shows the effectiveness of personalized recommendations or content in driving sales. |
Engagement Metrics | Metrics such as click-through rate (CTR) and time spent on site. | Helps assess how personalized experiences impact user interaction. |
How AI Personalization Enhances Customer Experience in Real-Time
AI-driven personalization allows businesses to tailor experiences based on individual customer behaviors, preferences, and interactions. By leveraging real-time data, companies can provide more relevant recommendations, content, and support, all while creating a more engaging and efficient user experience. This dynamic approach helps brands maintain a competitive edge by continuously adapting to customer needs and improving satisfaction across various touchpoints.
With the power of artificial intelligence, customer experiences can be personalized instantaneously. AI systems analyze vast amounts of data, processing it in real-time to predict and respond to customer actions. This ability to offer contextually relevant solutions as users interact with products or services ensures that every engagement is both meaningful and efficient. AI-based personalization is not just reactive but anticipatory, which makes it an essential tool for modern businesses.
Key Benefits of AI Personalization
- Enhanced Product Recommendations: AI algorithms analyze customer history and behavior to suggest items they are more likely to purchase, boosting sales and improving satisfaction.
- Real-Time Customer Support: AI-driven chatbots provide immediate assistance, reducing wait times and resolving issues quickly, ensuring a seamless customer experience.
- Personalized Marketing: Personalized ads and messages are delivered to users based on their preferences, leading to higher engagement and conversion rates.
"AI allows businesses to continuously adapt to customer needs, ensuring that each interaction feels tailored and relevant." – Tech Innovation Review
Real-Time Personalization in Action
Scenario | AI Application | Customer Benefit |
---|---|---|
Online Shopping | AI analyzes browsing behavior and purchase history to recommend products. | Customers receive personalized suggestions that match their tastes, increasing the likelihood of a sale. |
Customer Support | AI-powered chatbots handle inquiries instantly, providing solutions based on real-time analysis of customer issues. | Faster problem resolution and improved customer satisfaction. |
Email Marketing | AI segments users based on interests and past interactions to send customized offers. | Higher engagement rates and increased return on investment for marketing campaigns. |
"Real-time personalization not only improves the customer journey but also drives measurable business outcomes." – Digital Insights Magazine
Building Personalized Product Recommendations with AI Algorithms
Artificial intelligence (AI) has revolutionized the way companies engage with customers by enhancing the personalization of product suggestions. With AI-driven algorithms, businesses can analyze vast amounts of data, including user behavior, preferences, and demographic information, to provide tailored recommendations that resonate with each individual customer. These algorithms utilize various models, such as collaborative filtering, content-based filtering, and hybrid systems, to predict what products a customer might be interested in, increasing the likelihood of purchase.
Implementing AI for personalized recommendations involves creating models that continuously learn and adapt from user interactions. These models can predict future preferences by analyzing past behaviors like product views, clicks, and purchase history. The integration of deep learning techniques further improves the accuracy of these predictions by identifying complex patterns in user data. As a result, businesses can offer a unique shopping experience that keeps customers engaged and boosts conversion rates.
Key Techniques in Personalized Recommendations
- Collaborative Filtering: This approach uses user behavior data to recommend products based on the preferences of similar users.
- Content-Based Filtering: Recommends products by analyzing the characteristics of items the user has interacted with, such as categories, features, or descriptions.
- Hybrid Methods: Combines collaborative and content-based filtering to leverage the strengths of both techniques.
"AI-driven personalization is not just about recommending the right products but about creating a deeper connection with the customer by predicting what they will need next."
Challenges in Building Effective AI Recommendation Systems
- Data Quality: The success of AI algorithms depends heavily on the quality of input data, which must be clean and relevant.
- Scalability: Handling large volumes of data and ensuring that the recommendation system scales with the business is a key challenge.
- Bias and Diversity: Algorithms must be designed to avoid bias and ensure diverse product suggestions to appeal to a wide range of customers.
Performance Comparison of Algorithms
Algorithm | Strengths | Weaknesses |
---|---|---|
Collaborative Filtering | Effective for capturing user preferences based on behavior similarities. | Requires a large dataset to be accurate; may struggle with new users (cold start problem). |
Content-Based Filtering | Can recommend items based on specific item features, independent of other users. | May lead to narrow recommendations and lack diversity in suggestions. |
Hybrid Methods | Combines strengths of both collaborative and content-based filtering. | More complex to implement and maintain. |
Leveraging Data to Create Dynamic Content for Targeted Audiences
In today’s digital landscape, data has become a crucial tool for crafting personalized experiences that resonate with specific audience segments. By collecting and analyzing user behavior, preferences, and interactions, businesses can create tailored content that adapts to individual needs. The ability to adjust content in real time ensures higher engagement rates and improved customer satisfaction.
Dynamic content driven by data allows brands to stay relevant and competitive. It goes beyond just delivering generic messages and instead delivers specific, contextually relevant information to users at the right time. This strategy not only enhances user experience but also boosts conversions, as customers are more likely to engage with content that speaks directly to them.
Key Data Sources for Personalization
- Behavioral Data: Tracks user actions such as page visits, clicks, and time spent on specific content.
- Demographic Data: Includes age, location, interests, and other personal characteristics that help define audience segments.
- Transactional Data: Insights gathered from purchase history and customer interactions that indicate preferences and buying habits.
Benefits of Dynamic Content for Targeted Audiences
- Increased Relevance: Content can be customized to match user preferences and current interests.
- Improved Engagement: By delivering timely and relevant content, users are more likely to interact with the brand.
- Higher Conversion Rates: Personalization leads to a more direct connection with users, making them more likely to take desired actions.
"Personalized content can significantly increase user engagement and conversion rates by ensuring the right message is delivered to the right person at the right time."
Content Personalization Example
Segment | Content Type | Goal |
---|---|---|
New Visitors | Introduction video, overview of services | Educate about the brand |
Returning Customers | Discount offers, personalized recommendations | Encourage repeat purchases |
High-Engagement Users | Exclusive content, loyalty rewards | Strengthen brand loyalty |
Integrating AI Personalization into E-Commerce Platforms for Higher Conversions
Integrating AI-driven personalization into e-commerce platforms offers significant advantages in boosting user engagement and conversion rates. By leveraging machine learning and data analytics, businesses can provide tailored shopping experiences for each customer. This approach goes beyond simple product recommendations, enabling the platform to dynamically adjust to individual user preferences, behaviors, and even seasonal trends.
Through personalized experiences, e-commerce platforms can build stronger customer relationships, leading to increased brand loyalty and higher lifetime value. AI can help predict customer intent, optimize pricing strategies, and personalize product discovery, which results in a more seamless and effective shopping journey.
Key AI Personalization Techniques for E-Commerce
- Behavioral Targeting: Analyzing user actions such as browsing patterns, clicks, and past purchases to suggest relevant products in real-time.
- Dynamic Content Customization: Displaying personalized banners, promotions, and product categories based on user data and preferences.
- Predictive Analytics: Using historical data to forecast what products a user is likely to purchase, enhancing the recommendation system.
Steps for Effective Integration
- Data Collection: Gather relevant data points from various sources like browsing behavior, search queries, and demographic information.
- AI Model Training: Implement machine learning algorithms to analyze the collected data and create personalized experiences.
- Real-Time Personalization: Use AI to adjust content, offers, and product recommendations dynamically during a user’s session.
"AI personalization helps e-commerce platforms enhance their customer experience by making it more relevant, timely, and engaging, which directly translates into higher conversions."
Benefits of AI Personalization in E-Commerce
Benefit | Description |
---|---|
Increased Conversion Rates | Personalized experiences lead to a higher likelihood of users completing purchases. |
Improved Customer Retention | Tailored content encourages repeat visits and long-term brand loyalty. |
Optimized Marketing Spend | AI ensures that marketing efforts are focused on the most relevant customers, improving ROI. |
Leveraging Machine Learning to Enhance Customer Journeys and Retention
Modern businesses are increasingly turning to machine learning techniques to optimize customer experiences and improve retention rates. By analyzing vast amounts of customer data, machine learning algorithms can identify patterns and predict behavior, enabling companies to create personalized journeys that resonate with each individual. This approach not only enhances customer satisfaction but also encourages long-term loyalty by anticipating needs and preferences at every stage of the interaction.
Effective use of machine learning in customer journey optimization involves tracking and adapting to changes in behavior across various touchpoints. Machine learning models are capable of continuously refining strategies based on real-time data, making it easier to engage customers with highly relevant content, recommendations, and offers. The result is a more seamless and engaging experience that drives both acquisition and retention.
Key Strategies for Machine Learning Integration in Customer Retention
- Behavioral Segmentation: Machine learning analyzes customers' past actions to segment them into groups with similar needs, allowing for targeted campaigns.
- Predictive Analytics: Algorithms predict future actions, such as churn risk, helping companies take proactive measures to retain customers.
- Dynamic Personalization: Real-time data enables continuous adaptation of the customer journey, ensuring each interaction is highly relevant.
Techniques for Personalization and Retention Enhancement
- Personalized Product Recommendations: Using collaborative filtering, machine learning can suggest products that align with individual customer preferences.
- Targeted Messaging: By analyzing engagement patterns, businesses can tailor messaging to specific customer segments at the right time.
- Real-Time Interaction: AI-driven tools allow for immediate responses and offers, enhancing the customer experience during live interactions.
Important Note: Continuous feedback loops from machine learning models ensure that customer interactions are personalized and responsive, increasing the chances of long-term retention.
Data-Driven Insights for Optimizing Retention
Data Point | Machine Learning Application | Outcome |
---|---|---|
Purchase History | Behavioral Analysis | Better segmentation and targeted offers |
Engagement Metrics | Predictive Modeling | Early detection of churn risks |
Customer Feedback | Sentiment Analysis | Improved customer satisfaction and loyalty |
Addressing Privacy Challenges in AI-Powered Personalization
As AI continues to enhance user experiences through tailored content, products, and services, privacy concerns remain a major obstacle. The collection and analysis of personal data raise questions about the security of sensitive information, potential misuse, and the loss of control over personal privacy. Balancing the benefits of personalized experiences with the need to protect user privacy is essential for businesses and users alike.
While AI-driven systems offer significant advantages, their dependence on large datasets containing personal information necessitates a careful approach to managing privacy. Organizations must establish transparent practices and adhere to data protection regulations to ensure trust and accountability. By focusing on privacy-enhancing strategies, businesses can mitigate the risks and foster a secure environment for personalized experiences.
Key Approaches to Enhancing Privacy in AI Personalization
- Data Anonymization: Anonymizing data removes identifiable information, reducing the potential for misuse while still allowing for personalized insights.
- Transparency in Data Usage: Clearly communicating how data is collected, processed, and used helps build user trust and ensures that individuals are informed about their privacy rights.
- Consent Management: Users should have control over their data, with the ability to opt-in or opt-out of personalized services, ensuring informed consent at every step.
Additional strategies for ensuring privacy in AI systems include:
- Implementing strong encryption techniques for data storage and transmission.
- Using differential privacy techniques to analyze data without compromising individual user identities.
- Adopting privacy-preserving AI models that limit data exposure while still enabling accurate predictions.
"Protecting privacy is not only a legal obligation but a crucial aspect of maintaining user trust in personalized services powered by AI."
Comparison of Privacy Approaches in AI Personalization
Privacy Strategy | Benefit | Potential Challenge |
---|---|---|
Data Anonymization | Reduces risk of exposure while enabling personalized insights | May limit the depth of personalization |
Transparency | Builds trust and accountability with users | Requires ongoing communication and user education |
Consent Management | Empowers users to control their data | May reduce the volume of data available for personalization |
Measuring the Impact of AI-Driven Personalization
Evaluating the effectiveness of AI-powered personalization strategies is crucial for businesses aiming to understand the value and potential return on investment (ROI). By measuring the right metrics, organizations can optimize their personalization efforts and ensure they are achieving significant business outcomes. This involves tracking various data points, from user engagement to revenue generation, which directly correlate with the success of AI implementations. To accurately assess ROI, companies must go beyond surface-level results and focus on long-term value creation.
Key performance indicators (KPIs) are essential to determine how well AI personalization is delivering measurable benefits. These KPIs should be tailored to the business's specific objectives, whether it's improving customer retention, increasing sales conversions, or enhancing user satisfaction. Analyzing these metrics enables companies to fine-tune their strategies and better allocate resources for maximum impact.
Key Metrics to Measure ROI
- Customer Retention Rates: Increased retention suggests that personalized experiences are fostering customer loyalty.
- Conversion Rates: Personalization can boost conversions by presenting customers with more relevant product or content recommendations.
- Average Order Value (AOV): Personalized offers can increase the value of transactions by suggesting complementary or premium products.
- Customer Lifetime Value (CLV): Over time, personalized experiences can enhance the overall value of each customer relationship.
- Engagement Metrics: Tracking metrics like time spent on the platform or interaction frequency can indicate how effective the personalization is.
Approach to Calculating ROI
To calculate the ROI of AI personalization strategies, companies should follow a structured approach:
- Define Objectives: Clearly outline what the business aims to achieve with AI personalization (e.g., higher sales, improved customer experience).
- Establish Baselines: Gather data on current performance metrics to serve as a comparison point.
- Implement AI Solutions: Integrate AI-powered tools into the personalization process and track the associated changes.
- Measure Impact: After a set period, analyze key metrics such as conversion rates and customer engagement to quantify improvements.
- Calculate ROI: Subtract the investment costs from the gains achieved through AI personalization, then divide by the costs to determine ROI.
"ROI is not just about revenue; it’s about the long-term value AI personalization brings to customer relationships and brand loyalty."
Example of ROI Calculation
Metric | Before Personalization | After Personalization |
---|---|---|
Customer Retention | 60% | 75% |
Conversion Rate | 3% | 5% |
Average Order Value | $50 | $65 |