Marketing Ai Examples
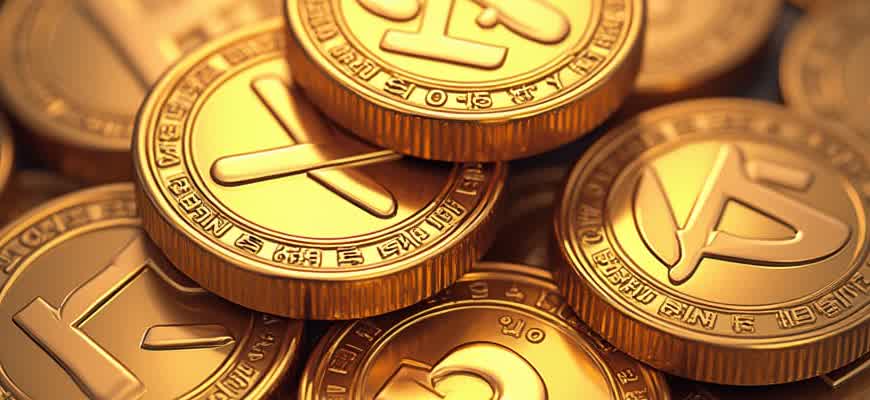
Artificial intelligence (AI) is transforming how companies engage with customers, personalize content, and optimize campaigns. Several AI-powered tools now enable businesses to enhance customer experiences and make data-driven decisions in marketing strategies.
Here are some practical applications of AI in the marketing industry:
- Customer Segmentation: AI algorithms analyze large datasets to identify customer segments based on behavior, demographics, and preferences.
- Chatbots and Virtual Assistants: AI-powered chatbots provide instant customer support and deliver personalized experiences through natural language processing (NLP).
- Predictive Analytics: AI uses past data to predict future consumer behavior and trends, helping marketers tailor their strategies accordingly.
AI is also streamlining content creation, optimizing advertising campaigns, and improving user experience across digital channels.
"AI-powered marketing tools enable businesses to make smarter decisions, saving time and resources while improving customer engagement." – Marketing Expert
Let's explore some specific AI technologies used in marketing:
AI Tool | Function | Example |
---|---|---|
Chatbots | Automate customer interactions and offer real-time assistance. | Intercom, Drift |
Content Personalization | Deliver tailored content based on user preferences and behavior. | Dynamic Yield, Optimizely |
Predictive Analytics | Anticipate future customer actions and trends. | HubSpot, Salesforce Einstein |
How Artificial Intelligence Enhances Customer Segmentation for Precise Campaigns
AI-driven solutions can significantly refine how businesses identify and target their ideal customers. Traditional segmentation methods based on basic demographics are being replaced with data-driven, AI-powered techniques that provide a deeper understanding of consumer behavior. By analyzing vast datasets, AI can create more granular customer profiles that enable businesses to craft personalized, high-impact marketing campaigns.
Through advanced machine learning models, companies can predict customer preferences, buying habits, and engagement patterns with greater accuracy. These insights allow brands to allocate resources effectively, reach the right people with tailored messaging, and ultimately increase conversion rates. AI's ability to process large amounts of unstructured data further adds to its power in identifying niche segments that may have been overlooked using traditional methods.
Key Benefits of AI for Customer Segmentation
- Data-Driven Segmentation: AI can analyze vast datasets, revealing hidden patterns and grouping customers based on behavior, interests, and other factors.
- Real-Time Insights: AI enables dynamic segmentation, constantly refining customer profiles as new data becomes available, allowing for up-to-date targeting.
- Predictive Analytics: AI models predict customer behavior, such as likelihood to purchase or churn, helping to optimize campaign timing and content.
Types of Segments AI Can Identify
- Behavioral Segmentation: Based on actions like browsing habits or past purchases.
- Demographic Segmentation: Age, gender, income, and other personal factors.
- Geographical Segmentation: Location-based insights for hyper-local targeting.
"AI-powered customer segmentation not only improves targeting accuracy but also enhances the overall customer experience by delivering content that is most relevant to each segment."
Example of AI-Based Segmentation Model
Segment | Criteria | Targeted Campaign |
---|---|---|
High-Value Customers | Frequent purchases, high average order value | Exclusive offers, loyalty rewards |
At-Risk Customers | Decreased engagement, previous churn risk | Re-engagement campaigns, discounts |
New Customers | First-time visitors or recent sign-ups | Welcome offers, introductory content |
AI-Driven Content Creation for Enhanced Engagement
Artificial intelligence has revolutionized the way brands create and distribute content. By automating the content creation process, businesses can focus more on strategy and optimization rather than spending valuable time on manual tasks. AI-powered tools can generate articles, blog posts, and social media updates with impressive speed, ensuring that companies stay relevant in a fast-paced digital world.
In addition to content creation, AI can also be employed to personalize user interactions, increasing engagement rates. Through data analysis, AI tools can understand customer preferences, allowing businesses to tailor their content to individual needs. This dynamic approach improves user experience and enhances overall engagement, making AI an indispensable asset in digital marketing.
Automating Content Creation with AI Tools
- Text Generation: AI can create high-quality articles, product descriptions, and ad copy with minimal human intervention, drastically reducing content production time.
- Social Media Posts: AI tools can schedule and create social media content based on trends, audience preferences, and engagement data, ensuring consistent online presence.
- Video and Image Content: AI-powered video editing software can generate videos from written content, while AI can also optimize images for better performance on different platforms.
AI Enhances User Interaction and Engagement
- Personalization: AI analyzes user behavior to create customized content that resonates with individual interests, improving click-through and conversion rates.
- Predictive Analytics: By understanding past behavior, AI can predict future actions, allowing marketers to anticipate customer needs and create relevant content in advance.
- Automated Responses: AI-driven chatbots and virtual assistants provide instant responses to customer inquiries, leading to increased satisfaction and engagement.
Key Benefits of AI Content Automation
Benefit | Description |
---|---|
Time Efficiency | AI can produce large volumes of content quickly, freeing up time for marketers to focus on strategy and optimization. |
Consistency | Automated tools ensure that content remains consistent across multiple platforms, maintaining brand voice and messaging. |
Scalability | AI allows businesses to scale their content production efforts without needing to increase resources or headcount. |
AI-driven content automation is not just about efficiency, but also about creating a personalized and engaging experience for users, which leads to higher conversion rates and improved brand loyalty.
Enhancing Lead Scoring with Predictive Analytics Powered by AI
In the modern marketing landscape, understanding which leads are most likely to convert is essential for optimizing sales efforts. AI-driven predictive analytics tools provide marketers with data-backed insights to score leads based on their potential for conversion. By analyzing historical data and behavior patterns, these tools help identify the most promising prospects, allowing businesses to prioritize their resources more effectively. This predictive approach enhances the efficiency of the sales pipeline and ensures that the marketing team focuses on the right audience segments.
Utilizing AI for lead scoring relies heavily on machine learning algorithms that analyze various data points such as past interactions, demographics, and online behavior. These insights allow for a more granular view of a lead’s likelihood to convert, compared to traditional manual scoring methods. Below are key components and benefits of incorporating AI-driven predictive analytics into lead scoring strategies:
Key Benefits of AI-Driven Lead Scoring
- Data-Driven Insights: AI models analyze large volumes of data to identify patterns and trends that human marketers might overlook.
- Real-Time Scoring: AI-powered tools continuously update lead scores in real time, adjusting to new interactions and behaviors.
- Improved Conversion Rates: By focusing on high-potential leads, businesses can improve conversion rates and optimize sales strategies.
- Increased Efficiency: Automation of lead scoring saves time and reduces manual errors, enabling marketing teams to focus on high-priority tasks.
How Predictive Analytics Improves Lead Scoring
Predictive analytics uses historical data to generate models that forecast the likelihood of a lead converting into a paying customer. By incorporating machine learning algorithms, marketers can refine their lead scoring process, making it more accurate and less reliant on subjective opinions. The AI system continuously learns from new data, improving the quality of its predictions over time.
"AI-driven lead scoring is not just about assigning scores, but about constantly refining the process to better align with evolving customer behavior and market conditions."
Key factors that AI models consider in predictive lead scoring include:
- Lead Demographics: Age, location, job title, and other personal information.
- Behavioral Data: Website visits, content downloads, email opens, etc.
- Engagement Level: Frequency and depth of interactions with marketing materials.
Example of a Predictive Lead Scoring Model
Lead Criteria | Score Weight | Predictive Value |
---|---|---|
Website Visits | 25% | Higher frequency of visits correlates with higher likelihood to convert. |
Email Engagement | 30% | Frequent clicks and opens signal strong interest in products or services. |
Lead Demographics | 20% | Specific demographic groups have higher conversion rates in certain industries. |
Past Purchases | 25% | Previous buyers are more likely to engage in future transactions. |
Enhancing Email Campaigns with AI-driven Personalization
Email marketing is an essential tool for engaging customers and driving sales. However, as inboxes become more crowded, it is increasingly important to create personalized experiences that stand out. Artificial intelligence offers the ability to leverage customer data and deliver content tailored to each individual. By incorporating AI-driven techniques into email marketing strategies, businesses can increase open rates, improve engagement, and boost customer retention.
AI allows marketers to analyze customer behaviors, preferences, and interactions with previous emails to create highly customized messages. Through the use of machine learning and predictive analytics, businesses can automate segmentation, subject line optimization, and content recommendations, making each email feel unique to the recipient. This level of personalization can significantly enhance the effectiveness of email campaigns.
Key AI Personalization Strategies
- Dynamic Content Personalization: AI can tailor email content, including images, text, and offers, based on user data. For instance, if a customer has shown interest in specific products, the email content will reflect those preferences.
- Predictive Analytics: By analyzing past behaviors, AI can predict when a customer is most likely to engage, optimizing send times for maximum open rates.
- Automated Segmentation: AI algorithms can categorize customers into dynamic segments based on their interactions with previous campaigns, allowing for more relevant targeting.
Benefits of AI Personalization in Email Marketing
- Increased Engagement: Personalized emails tend to capture attention more effectively, leading to higher click-through rates and conversions.
- Time Efficiency: Automation tools powered by AI reduce the need for manual intervention, allowing marketing teams to focus on strategic planning rather than repetitive tasks.
- Enhanced Customer Experience: Personalized messages make recipients feel valued, improving brand loyalty and customer retention.
"AI personalization takes the guesswork out of email marketing, enabling brands to deliver content that resonates with each individual customer."
Example of AI-Powered Email Campaign
Customer Data | Email Content |
---|---|
Recent Purchases: Sneakers, Running Gear | Subject: "New Running Shoes You Might Love!" Body: "Based on your recent purchase, we thought you'd like these new arrivals in running shoes. Don't miss out!" |
Browsing Behavior: Activewear, Fitness Accessories | Subject: "Top Fitness Gear for Your Workout Routine" Body: "Check out the latest collection of fitness accessories tailored to your needs!" |
AI-Driven Social Media Monitoring for Instant Analysis
With the rapid growth of digital platforms, brands are increasingly turning to advanced technologies to track real-time conversations and trends on social media. AI-powered tools are now capable of monitoring millions of posts across various platforms, providing instant insights into consumer sentiments, brand perceptions, and industry developments. By leveraging machine learning and natural language processing, these tools can detect emerging trends, potential PR crises, and shifts in public opinion almost as they happen.
AI-based social listening tools help businesses make data-driven decisions by analyzing vast amounts of unstructured data. These tools not only process the text but also recognize contextual nuances such as tone, sentiment, and emotional triggers, offering a deeper understanding of online conversations. Real-time feedback helps marketers and brand managers react swiftly to customer inquiries, manage brand reputation, and refine marketing strategies.
Key Benefits of AI Social Listening
- Immediate Insights: AI tools provide instant data analysis, enabling brands to act swiftly on new developments.
- Sentiment Analysis: Detects positive, neutral, or negative sentiments in posts, helping businesses gauge public opinion.
- Competitor Monitoring: Tracks competitors' social media activity, offering insights into their strategies and audience reactions.
- Trend Identification: Uncovers emerging topics and hashtags, guiding content creation and campaign planning.
How AI Listening Tools Work
- Data Collection: AI tools aggregate posts from various platforms like Twitter, Facebook, Instagram, and more.
- Data Processing: The collected data is filtered, and AI algorithms analyze text for keywords, sentiment, and context.
- Real-Time Alerts: Users receive notifications on shifts in sentiment or emerging trends, allowing quick responses.
"AI-powered listening enables brands to stay ahead of trends and quickly mitigate risks before they escalate into major issues."
Example Metrics from AI Listening Tools
Metric | Example Value |
---|---|
Sentiment Score | +87% Positive |
Trending Hashtags | #SummerSale, #BrandName |
Brand Mentions | 4,500 Mentions in 24 hours |
Optimizing Pricing with AI-Driven Dynamic Strategies
AI-based pricing models are transforming the way businesses approach pricing decisions. Through sophisticated algorithms, businesses can now analyze vast amounts of data in real-time, enabling them to adjust prices dynamically based on demand, competitor behavior, and customer preferences. This flexibility ensures that prices are always aligned with market conditions, maximizing both revenue and customer satisfaction.
With AI, pricing strategies are no longer static. They evolve continuously, driven by data insights, customer trends, and competitive landscape changes. AI algorithms assess factors like consumer purchasing behavior, seasonal trends, and external market influences to make pricing decisions that would be impossible to achieve manually.
Key Elements of AI-Powered Dynamic Pricing
- Data Analysis: AI analyzes historical and real-time data to predict demand fluctuations.
- Competitor Monitoring: AI monitors competitor prices and adjusts accordingly to remain competitive.
- Customer Segmentation: AI can tailor prices to specific customer segments based on preferences and behavior.
- Demand Forecasting: AI forecasts future demand and adjusts prices proactively to optimize sales.
Here’s how the AI-powered pricing algorithm typically works:
Step | Action | Outcome |
---|---|---|
1 | Data Collection | Gather historical and real-time data on prices, demand, and competitors. |
2 | Demand Prediction | AI predicts future demand based on current market trends. |
3 | Price Adjustment | Prices are adjusted dynamically to reflect predicted demand and competitor pricing. |
4 | Performance Analysis | AI analyzes sales and adjusts strategy based on results. |
AI-based dynamic pricing systems ensure businesses can maintain competitiveness while optimizing profits, making them essential for companies in fast-moving markets.
Enhancing Ad Campaign Performance with AI-Driven A/B Testing
Artificial Intelligence (AI) has revolutionized digital marketing by enabling more precise and efficient optimization of advertising strategies. One of the most powerful applications is AI-based A/B testing, which allows marketers to evaluate multiple ad variations in real-time and identify the most effective elements for their target audience. By automating the testing process, AI removes the guesswork and accelerates decision-making, leading to higher conversion rates and improved ROI.
AI-driven tools can analyze large amounts of data from A/B tests, identifying patterns that humans might miss. By utilizing machine learning algorithms, these tools optimize ad creatives, copy, targeting strategies, and even bidding in real time. This advanced approach not only enhances the speed of testing but also allows for more granular insights that help fine-tune campaign strategies for maximum effectiveness.
Key Benefits of AI in A/B Testing
- Real-Time Data Processing: AI processes large datasets instantly, allowing for quicker adjustments and optimizations.
- Precision in Targeting: Machine learning algorithms help refine audience segmentation, ensuring ads reach the most relevant users.
- Cost Efficiency: By identifying the best-performing ads early, AI minimizes wasted spend and maximizes campaign ROI.
How AI Optimizes A/B Test Results
- Dynamic Ad Creation: AI continuously generates and tests new ad variants based on user behavior and engagement metrics.
- Predictive Analytics: AI can predict the success of an ad variation before it reaches a large audience, reducing the need for extensive testing.
- Performance Measurement: AI evaluates metrics such as click-through rate (CTR), conversion rate, and engagement to determine which elements drive results.
AI-based A/B testing enables continuous optimization without the need for constant human intervention, making the process more efficient and accurate.
AI-Driven A/B Testing in Action
Ad Element | AI Optimization | Impact |
---|---|---|
Headline | AI tests multiple headline variations and selects the one with the highest engagement. | Improved click-through rate (CTR) due to more compelling messaging. |
Imagery | AI analyzes user reactions to different images and chooses the most effective visuals. | Higher engagement and conversion rates driven by more relevant imagery. |
Call to Action | AI identifies the most effective call-to-action phrase based on user preferences. | Increased conversions through more persuasive CTAs. |
Creating AI-Powered Customer Support Chatbots
AI-driven chatbots have become an essential tool for businesses looking to enhance their customer service. By utilizing natural language processing (NLP) and machine learning, these systems are capable of handling customer inquiries, providing timely responses, and resolving common issues efficiently. Chatbots can simulate human-like conversations, allowing businesses to automate customer interactions and improve response time.
The integration of AI tools into customer support systems reduces the workload for human agents, enabling them to focus on more complex queries. Moreover, AI chatbots are available 24/7, ensuring that customers receive help at any time. This constant availability enhances user satisfaction and loyalty, offering a competitive edge in the market.
Steps to Build an Effective AI Customer Support Chatbot
- Define Purpose: Clearly determine the primary function of the chatbot, such as answering FAQs or providing troubleshooting assistance.
- Select Tools: Choose the right AI tools and platforms for chatbot development, considering factors like ease of integration and NLP capabilities.
- Train the Model: Input various customer queries and responses to help the chatbot understand and generate accurate replies.
- Implement Escalation Protocols: Ensure that the chatbot can transfer complex cases to human agents when necessary.
- Monitor and Optimize: Regularly analyze chatbot performance and refine its responses based on user feedback.
AI chatbots can handle a large volume of inquiries simultaneously, offering faster solutions and improving overall customer experience.
Benefits of Using AI in Customer Support
Benefit | Description |
---|---|
Cost Reduction | AI chatbots can handle many queries without human intervention, reducing the need for large customer service teams. |
Increased Efficiency | By automating repetitive tasks, chatbots free up human agents to handle more complex issues. |
Scalability | AI chatbots can handle an unlimited number of queries simultaneously, making them ideal for businesses with fluctuating demand. |