7 Ai Saas Ideas
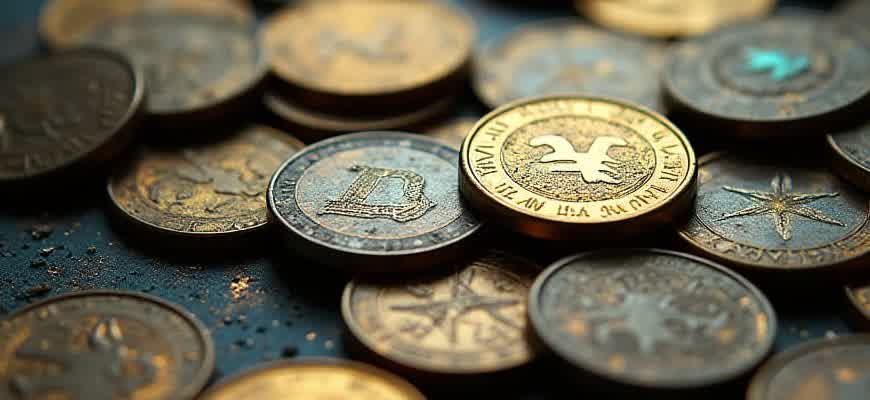
The rapid evolution of artificial intelligence has opened up numerous opportunities for SaaS (Software as a Service) applications. Below are seven compelling ideas that could revolutionize industries and create new market niches:
- AI-Driven Customer Support Automation: Leverage AI chatbots and virtual assistants to provide real-time customer service with minimal human intervention.
- Predictive Analytics for Business Intelligence: Offer predictive tools to help businesses forecast trends, consumer behavior, and inventory needs based on data analysis.
- Personalized Marketing Platforms: Build AI-powered platforms that tailor advertising and content to individual preferences using machine learning algorithms.
Each of these ideas can be adapted to fit a wide range of industries. Here's a brief overview of the key features that could make each solution successful:
AI SaaS Idea | Core Feature | Potential Industry Impact |
---|---|---|
AI-Driven Customer Support | 24/7 automated customer interactions | Improves customer satisfaction, reduces operational costs |
Predictive Analytics | Real-time forecasting and trend analysis | Enhances decision-making, optimizes resource allocation |
Personalized Marketing Platforms | Custom content and ad targeting | Boosts conversion rates, increases user engagement |
"AI is not just about automating processes; it's about empowering businesses to make smarter decisions and enhance customer experience."
7 AI SaaS Ideas to Accelerate Business Expansion
As businesses continue to navigate the digital age, leveraging artificial intelligence (AI) through SaaS platforms has become a game-changer. These AI-powered services enable companies to scale operations, enhance decision-making, and improve customer experience. Below are seven innovative AI-driven SaaS concepts that can fuel growth in a variety of industries.
AI SaaS solutions not only streamline complex processes but also provide businesses with actionable insights and automation capabilities. From predictive analytics to personalized customer support, the opportunities are limitless for companies looking to stay ahead of the curve.
1. AI-Powered Customer Segmentation Tools
Customer segmentation is crucial for targeted marketing and sales strategies. AI-driven platforms can analyze vast amounts of customer data to identify specific patterns, behaviors, and preferences. These tools help businesses create highly personalized marketing campaigns, resulting in higher conversion rates.
- Utilizes machine learning to segment customers based on behavior.
- Enhances marketing strategies with data-driven insights.
- Improves targeting precision and increases ROI.
2. Automated Content Generation Platforms
AI can transform content creation by automating the writing process. Platforms powered by AI algorithms can generate blog posts, social media content, and even personalized email campaigns, reducing the time and cost spent on content production.
"AI can now write content that feels human, saving time and resources while maintaining high quality."
3. AI-Based Predictive Analytics for Supply Chain Optimization
Predictive analytics driven by AI can significantly enhance supply chain management. These platforms forecast demand, optimize inventory, and predict potential disruptions. With real-time insights, businesses can make proactive decisions and reduce operational costs.
- Forecasts product demand with high accuracy.
- Identifies potential supply chain bottlenecks in advance.
- Reduces excess inventory and associated costs.
4. AI-Powered Chatbots for 24/7 Customer Support
AI chatbots are revolutionizing customer support by providing instant responses to inquiries at any time of day. These chatbots are capable of handling a wide range of customer issues, from product queries to technical troubleshooting, improving overall customer satisfaction.
Benefit | Impact |
---|---|
24/7 Availability | Improves customer experience with immediate support. |
Scalable Support | Can handle thousands of queries simultaneously. |
5. AI-Driven Fraud Detection Systems
With increasing cyber threats, AI can assist businesses in identifying fraudulent activities in real-time. These systems use machine learning to detect unusual patterns and flag potential threats before they cause significant damage.
6. AI-Powered HR Analytics Platforms
AI tools can streamline the hiring process by analyzing resumes, conducting sentiment analysis on interviews, and even predicting candidate success within a company. This results in more efficient recruitment and better talent management.
7. AI-Enhanced Personalization Engines
Personalized user experiences are key to customer retention. AI-powered platforms use behavioral data to recommend products, services, or content tailored to individual preferences, increasing customer satisfaction and sales.
How AI Chatbots Can Streamline Customer Support
Automating customer support through AI-powered chatbots can significantly enhance the efficiency and scalability of businesses. By handling routine inquiries, these chatbots reduce response time and free up human agents to focus on more complex issues. AI chatbots not only provide 24/7 service but also adapt to various customer needs, making them an indispensable tool for improving user experience and operational efficiency.
Incorporating AI chatbots into your support strategy requires understanding the core components that drive their success. These systems rely on natural language processing (NLP) and machine learning to provide accurate responses and handle different customer interactions, ensuring that issues are addressed promptly and effectively.
Key Benefits of AI Chatbots in Customer Support
- Instant Response Time: AI chatbots can provide immediate assistance, significantly reducing wait times for customers.
- 24/7 Availability: Customers can receive support anytime, improving satisfaction and engagement.
- Cost Efficiency: Reducing the need for human intervention for simple tasks allows companies to allocate resources more effectively.
- Personalization: Advanced AI systems can tailor responses based on customer history, preferences, and previous interactions.
Steps to Implement AI Chatbots for Customer Support
- Define Use Cases: Identify the types of queries and tasks that the chatbot will handle, such as FAQs, account issues, or troubleshooting.
- Integrate with Existing Systems: Ensure the chatbot integrates seamlessly with CRM tools, helpdesk systems, and other platforms to access necessary data.
- Train the AI: Use historical chat logs and customer interactions to train the chatbot, improving its ability to provide accurate and helpful responses.
- Monitor and Optimize: Regularly analyze chatbot performance and make adjustments based on feedback and new customer needs.
AI-powered chatbots can handle up to 80% of routine customer interactions, significantly reducing operational costs and enhancing the overall customer experience.
Common AI Chatbot Features to Include
Feature | Description |
---|---|
Natural Language Understanding (NLU) | Allows the bot to comprehend and respond to customer queries in a human-like manner. |
Context Awareness | The bot can remember previous interactions to provide more personalized and relevant responses. |
Multi-Channel Support | Chatbots can operate across various platforms, including websites, social media, and messaging apps. |
Creating Tailored Marketing Campaigns with AI SaaS Platforms
AI-powered SaaS platforms provide businesses with the ability to design and implement highly personalized marketing strategies. By leveraging advanced algorithms, these tools can analyze customer data, segment audiences, and predict behaviors to create campaigns that resonate with individual preferences. With this capability, marketers can deliver relevant content, offers, and experiences, significantly improving conversion rates and customer loyalty.
AI-driven platforms also enable real-time optimization of campaigns, ensuring that messaging evolves based on user interactions. These platforms are particularly valuable in an era where customers expect customized communication. The use of predictive analytics, behavioral tracking, and dynamic content generation makes these tools indispensable for modern marketing teams.
Key Features of AI SaaS Tools for Personalized Marketing
- Audience Segmentation: AI tools can automatically categorize customers into segments based on behaviors, interests, and demographics.
- Dynamic Content Delivery: The ability to adjust content in real-time based on user interactions and preferences.
- Predictive Analytics: Forecasting customer behaviors and trends to deliver timely, targeted offers.
- Multichannel Integration: Seamlessly connecting marketing efforts across various platforms, from email to social media.
Benefits of Using AI in Marketing Campaigns
- Enhanced Customer Engagement: Personalized experiences drive higher engagement rates and deeper customer relationships.
- Cost Efficiency: Optimized campaigns reduce wasted spend by targeting only the most relevant audience segments.
- Improved ROI: Data-driven decisions lead to better targeting, resulting in a higher return on marketing investments.
Example of AI-Driven Campaign Process
Step | Action | AI Tool Feature |
---|---|---|
1 | Customer Data Collection | Behavioral Analytics |
2 | Segmentation | Machine Learning Algorithms |
3 | Personalized Content Delivery | Real-Time Content Adjustment |
4 | Campaign Optimization | Predictive Analytics |
Important: AI-driven marketing tools not only automate processes but also continuously refine their strategies to meet evolving customer needs.
Leveraging AI for Real-Time Data Analysis in SaaS Solutions
Integrating artificial intelligence (AI) into Software-as-a-Service (SaaS) platforms has the potential to revolutionize the way businesses analyze real-time data. AI-powered algorithms can quickly process vast amounts of information, offering insights that would take human analysts hours or even days to uncover. By leveraging AI, SaaS providers can enable clients to make data-driven decisions in real time, improving operational efficiency and delivering more personalized services.
Real-time data analysis is crucial in many industries, especially in fields such as finance, healthcare, and e-commerce, where immediate insights can lead to competitive advantages. By embedding AI into SaaS solutions, companies can automate complex data workflows, identify trends, and offer predictive analytics–all in a fraction of the time traditionally required. This not only accelerates decision-making but also enhances user experience by delivering actionable insights on-demand.
Key Benefits of AI-Driven Real-Time Data Analysis
- Speed and Efficiency: AI can process large datasets in seconds, enabling real-time insights and quicker decision-making.
- Predictive Capabilities: AI algorithms can identify patterns and forecast future trends, giving users an edge in planning and strategy.
- Scalability: AI-based tools can handle increasing data volumes without compromising performance, ensuring the SaaS solution grows with the business.
How AI Enhances Real-Time Data Analysis in SaaS Platforms
- Data Aggregation and Cleaning: AI automates the process of gathering, cleaning, and organizing data, ensuring that the analysis is accurate and actionable.
- Real-Time Insights: By continuously analyzing incoming data streams, AI can provide up-to-the-minute reports, helping businesses stay agile.
- Advanced Analytics: Machine learning models within AI can recognize anomalies, perform sentiment analysis, and provide in-depth insights that go beyond traditional analytics.
"The ability to analyze data in real-time empowers businesses to adjust strategies instantly, leading to enhanced customer satisfaction and better financial outcomes."
Practical Applications in SaaS
Industry | AI Application | Benefit |
---|---|---|
Healthcare | Real-time patient monitoring and predictive diagnostics | Improved patient outcomes through faster interventions |
E-Commerce | Personalized product recommendations based on real-time user behavior | Increased conversion rates and customer satisfaction |
Finance | Fraud detection and risk management | Reduced financial loss and enhanced security |
Building Scalable AI-Powered Content Creation Platforms
Creating a scalable platform for content generation using AI technologies can revolutionize industries ranging from marketing to media. By automating content creation, businesses can deliver high-quality output consistently and at scale. Such platforms typically leverage Natural Language Processing (NLP) and deep learning models to generate personalized, engaging content that resonates with various audiences.
The key to scalability lies in designing a robust infrastructure that can handle increasing volumes of requests while maintaining content quality. This is achieved by integrating advanced algorithms with cloud-based resources to ensure both speed and flexibility in content generation. As platforms grow, the system should also adapt, enabling continuous learning from user feedback and content performance.
Key Components of Scalable Content Creation Platforms
- AI Models: Deep learning models and transformers that generate high-quality text across various formats.
- Data Integration: Seamless integration with databases and external APIs for real-time content updates.
- Content Personalization: Ability to tailor content based on user preferences and behavior.
- Performance Analytics: Monitoring and analyzing generated content to refine algorithms.
Challenges to Overcome
- Data Quality: Ensuring high-quality, diverse data sets for model training is essential.
- Content Relevance: Maintaining relevance and accuracy in generated content.
- Computational Costs: Managing cloud resources efficiently to handle growing demand.
"The success of an AI-driven content creation platform hinges on its ability to scale without sacrificing content quality or speed."
Performance Metrics for AI Content Platforms
Metric | Description | Importance |
---|---|---|
Response Time | Time taken to generate content after a request. | Critical for user satisfaction and real-time applications. |
Content Accuracy | Alignment of generated content with user intent and context. | Ensures the platform provides valuable and relevant outputs. |
Scalability | System’s ability to handle growing demand and requests. | Vital for maintaining platform performance as it expands. |
Developing AI-Powered Predictive Analytics for Sales Optimization
AI-driven predictive analytics can significantly enhance the sales process by analyzing large sets of data to forecast trends, optimize strategies, and identify potential sales opportunities. By leveraging machine learning models, businesses can predict future customer behaviors, segment prospects, and improve targeting precision. This approach goes beyond traditional methods by providing data-driven insights into customer preferences and the likelihood of conversion, offering sales teams actionable intelligence to adjust their efforts in real-time.
The core advantage of AI in sales optimization is its ability to process and analyze complex datasets faster and more accurately than human teams. The predictive models can identify patterns in customer interactions, buying history, and engagement levels, helping to optimize sales tactics and forecast revenue growth. By integrating these AI insights into the sales workflow, organizations can enhance decision-making, reduce manual effort, and drive better outcomes with minimal resources.
Key Benefits of Predictive Sales Analytics
- Increased Lead Conversion: AI models analyze historical data to identify high-potential leads, improving conversion rates.
- Optimized Pricing Strategies: Predictive analytics can recommend dynamic pricing models based on market conditions and competitor behavior.
- Enhanced Customer Targeting: Machine learning helps segment customers with a high probability of engagement, ensuring better targeting and personalized offers.
How AI Optimizes Sales Processes
- Data Collection: Gather customer interactions, sales transactions, and behavioral data across multiple touchpoints.
- Model Training: Use historical data to train predictive models for lead scoring, sales forecasting, and churn prediction.
- Real-time Insights: Provide sales teams with actionable insights based on real-time data analysis for timely intervention.
- Continuous Improvement: The model learns and adapts over time, refining predictions and strategies for ongoing optimization.
AI-powered sales tools not only save time but also enhance the accuracy of sales forecasts, allowing teams to make better decisions based on data-driven insights rather than intuition alone.
Sales Optimization with Predictive Analytics: A Case Study
Company | Problem | Solution | Outcome |
---|---|---|---|
Company A | Low conversion rate | Implemented AI predictive models to analyze customer behavior | 25% increase in lead conversion rate |
Company B | Poor sales forecasting | Used machine learning for accurate revenue predictions | 30% improvement in forecasting accuracy |
Integrating AI-Driven Fraud Prevention into SaaS Solutions
Incorporating AI-based fraud detection into SaaS products enhances security by automating the identification of suspicious activities. It allows businesses to respond faster and more accurately to potential threats. Leveraging machine learning algorithms, these systems analyze vast amounts of transactional and behavioral data to detect unusual patterns that may indicate fraud, without relying solely on traditional methods. This approach ensures that organizations can prevent fraud before it becomes a significant problem.
The integration of AI in fraud detection also offers scalability, adapting to the growing volume of data and evolving fraud tactics. With this technology, SaaS providers can offer clients a robust security system, which continuously improves its detection accuracy by learning from new data inputs. The solution can be seamlessly incorporated into existing platforms, offering immediate protection while reducing manual intervention.
Key Benefits of AI-Powered Fraud Detection
- Real-time Monitoring: AI systems monitor transactions in real-time, flagging suspicious behavior as it occurs.
- Scalability: Machine learning models evolve, making it easier to handle large data sets and adjust to new fraud techniques.
- Reduced False Positives: Advanced algorithms refine detection accuracy, minimizing false alerts and improving overall efficiency.
- Cost Reduction: Automating fraud detection reduces the need for large, specialized security teams.
How AI Fraud Detection Works
- Data Collection: Gather data from various sources, such as transaction history, user behavior, and external databases.
- Pattern Recognition: AI systems analyze this data to identify typical patterns and flag anomalies.
- Continuous Learning: The system uses feedback loops to improve detection capabilities by learning from past fraudulent incidents.
- Risk Assessment: The AI assigns a risk score to transactions, prioritizing those that require immediate attention.
Example Use Case
Scenario | Traditional Method | AI-Powered Approach |
---|---|---|
Unusual login location | Manual verification by a support team | AI flags and automatically blocks or requests additional verification |
Multiple failed payment attempts | Alert generated, but often ignored | AI triggers an alert and evaluates risk based on behavior and historical data |
Integrating AI into fraud detection systems not only enhances security but also allows businesses to stay ahead of evolving threats, offering a future-proof solution to protect sensitive data and transactions.